O marketing é uma das áreas mais importantes de uma empresa, pois é responsável por atrair e manter clientes, gerar receita e fortalecer a marca. Para alcançar esses objetivos, existem diversas estratégias de marketing que podem ser adotadas.
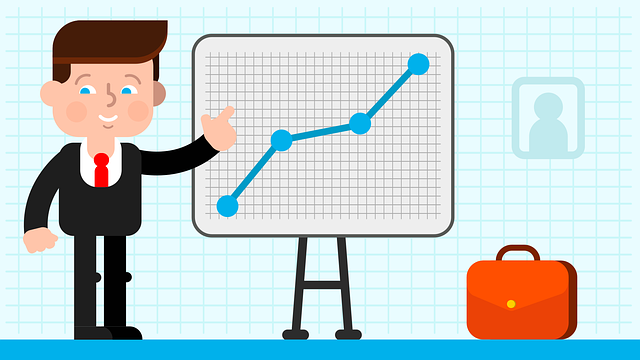
Neste artigo, vamos abordar as 4 principais estratégias de marketing que podem ser utilizadas pelas empresas para se destacar no mercado e obter sucesso em suas atividades. Acompanhe conosco e descubra como aplicar essas estratégias em seu negócio.
Pilares essenciais do marketing.
O marketing é uma atividade essencial para qualquer empresa que deseja se destacar no mercado e conquistar mais clientes. Existem quatro pilares essenciais do marketing que precisam ser considerados para que uma estratégia seja bem-sucedida:
- Produto: o produto ou serviço oferecido pela empresa precisa atender às necessidades e expectativas dos clientes. Além disso, é importante que o produto seja diferenciado e tenha algum valor agregado que o destaque da concorrência.
- Preço: o preço do produto ou serviço deve ser justo e competitivo em relação ao mercado. É importante considerar os custos de produção, as despesas da empresa e a margem de lucro desejada para definir o preço ideal.
- Promoção: a promoção é a forma como a empresa divulga o seu produto ou serviço para o público-alvo. É importante escolher os canais de comunicação adequados e elaborar uma mensagem clara e persuasiva para atrair clientes.
- Praça: a praça se refere ao local onde o produto ou serviço será comercializado. É importante escolher os canais de distribuição adequados e garantir que o produto esteja disponível nos locais em que os clientes procuram por ele.
Considerar esses quatro pilares essenciais do marketing é fundamental para que uma empresa possa elaborar uma estratégia eficaz e conquistar mais clientes. Cada um desses elementos precisa ser cuidadosamente planejado e executado para que a empresa possa alcançar seus objetivos de negócio.
Tipos de marketing: uma visão geral
O marketing é uma área de grande importância para as empresas, pois é por meio dele que elas conseguem atrair e fidelizar clientes. Existem diversos tipos de marketing que podem ser utilizados, cada um com suas características e objetivos específicos. Neste artigo, vamos apresentar uma visão geral sobre os tipos de marketing mais comuns e suas principais estratégias.
1. Marketing de produto
O marketing de produto é focado em promover e vender um produto específico, destacando suas características e benefícios para o consumidor. As principais estratégias de marketing de produto incluem o lançamento de novos produtos, o desenvolvimento de embalagens atraentes, a realização de promoções e descontos, e a criação de campanhas publicitárias que destaquem os diferenciais do produto.
2. Marketing de relacionamento
O marketing de relacionamento tem como objetivo criar e manter um relacionamento duradouro entre a empresa e seus clientes, com base na confiança e na fidelidade. As principais estratégias de marketing de relacionamento incluem o atendimento personalizado, a oferta de programas de fidelidade e recompensas, o envio de newsletters e e-mails marketing, e a realização de eventos exclusivos para clientes.
3. Marketing digital
O marketing digital é uma das áreas que mais tem crescido nos últimos anos, pois permite que as empresas alcancem um grande público de forma rápida e eficiente. As principais estratégias de marketing digital incluem a criação de sites e blogs, a utilização de redes sociais, a criação de conteúdo relevante, a realização de campanhas de e-mail marketing e a utilização de técnicas de SEO (Search Engine Optimization) para melhorar o posicionamento nos resultados de busca.
4. Marketing de experiência
O marketing de experiência tem como objetivo proporcionar uma experiência única e marcante para o consumidor, criando uma ligação emocional com a marca. As principais estratégias de marketing de experiência incluem a realização de eventos e experiências exclusivas, a criação de ambientes diferenciados nas lojas, a utilização de tecnologias interativas, e a oferta de serviços diferenciados que vão além do produto em si.
Os 4 As do Marketing.
O conceito dos 4 As do Marketing foi criado por Jerome McCarthy em 1960 e é uma ferramenta importante para a elaboração de estratégias de marketing. Os 4 As do Marketing são:
- Produto (Product): refere-se ao conjunto de bens e serviços oferecidos pela empresa aos seus consumidores. É importante que o produto atenda às necessidades e desejos dos clientes e esteja de acordo com as tendências do mercado.
- Preço (Price): é o valor monetário que o consumidor paga pelo produto. O preço deve ser justo e competitivo em relação aos concorrentes, levando em consideração os custos de produção e a margem de lucro desejada pela empresa.
- Praça (Place): refere-se aos canais de distribuição utilizados pela empresa para disponibilizar o produto ao consumidor. É importante que o produto esteja disponível nos locais onde o cliente costuma procurar e que a empresa tenha uma logística eficiente para distribuição.
- Promoção (Promotion): diz respeito às estratégias de comunicação utilizadas pela empresa para divulgar o produto e atrair clientes. Isso pode incluir propagandas, promoções, relações públicas, entre outras ações.
Os 4 As do Marketing são uma ferramenta importante para garantir o sucesso de uma estratégia de marketing, permitindo que a empresa elabore um plano completo que leve em consideração todos os aspectos do produto, preço, praça e promoção.
Estratégias de Marketing Essenciais.
As estratégias de marketing essenciais são fundamentais para o sucesso de qualquer negócio. Elas incluem:
- Segmentação de mercado: identificação e divisão do mercado em grupos específicos de consumidores com características e necessidades semelhantes.
- Posicionamento de marca: definição da imagem e da personalidade da marca no mercado, com o objetivo de diferenciá-la e torná-la única.
- Marketing de conteúdo: criação e distribuição de conteúdo relevante e valioso para atrair e engajar o público-alvo.
- Inbound marketing: estratégia que visa atrair, converter, fechar e encantar clientes por meio de conteúdo útil e relevante.
Essas estratégias são essenciais para alcançar e conquistar o público-alvo, aumentar as vendas e o reconhecimento da marca no mercado, além de construir uma relação de confiança e fidelidade com os clientes.
Conclusão
As quatro estratégias de marketing discutidas neste artigo – produto, preço, promoção e distribuição – são fundamentais para qualquer empresa que deseja alcançar o sucesso em seus negócios. É importante lembrar que essas estratégias devem ser adaptadas às necessidades e objetivos de cada empresa, levando em consideração o mercado em que atua e o público-alvo que deseja alcançar.
Ao utilizar essas estratégias de forma eficaz, é possível construir uma marca forte e aumentar a fidelidade dos clientes. As quatro estratégias de marketing mais comuns são: o marketing de produto, o marketing de preço, o marketing de promoção e o marketing de distribuição.
Cada uma dessas estratégias tem um papel importante na construção da marca e no sucesso de uma empresa. O marketing de produto envolve a criação de um produto de alta qualidade que atenda às necessidades dos clientes.
O marketing de preço visa definir preços competitivos que sejam atraentes para o público-alvo. O marketing de promoção é responsável pela divulgação da marca e seus produtos através de publicidade e outras estratégias de comunicação.
Por fim, o marketing de distribuição é responsável por garantir que os produtos cheguem aos clientes de maneira eficiente e conveniente. Ao adotar as quatro estratégias de marketing, as empresas podem aumentar sua visibilidade, ampliar sua base de clientes e alcançar o sucesso no mercado.